The increased availability of data for research has paradoxically contributed to scientific misinformation. When people lack the knowledge to analyze data critically, data can be misused and misinterpreted.
While easy access to information is helpful, it also allows bad analysis to spread unchecked. The problem is compounded when poorly done studies are published in scientific journals, leading the lay public to believe they are “scientifically validated.”
The peer review crisis
Reputable scientific publications use a peer review system. Experts in the field of the article evaluate the studies before publication to ensure their quality. However, changes in publishers’ business models are undermining this system. Many scientific journals now prioritize profit, accepting poorly written articles in exchange for paying publishing fees. The more articles they publish, the more they earn, without worrying about quality.
These journals perform superficial reviews, calling in reviewers without adequate expertise to reread the articles. This results in the publication of studies with serious errors, which are treated as reliable science.
One example is the article “Evaluation of post-COVID mortality risk in cases classified as severe acute respiratory syndrome in Brazil: a longitudinal study for medium and long term,” published in Frontiers in Medicine in December 2024. The reviewers of this article were not experts in the field, nor did they have any experience in the epidemiology of COVID-19 in Brazil.
The paper claims that COVID-19 vaccines increase the risk of non-COVID-related death one year after infection. Surprisingly, the only criterion used to define “non-COVID-related” deaths was that they occurred more than three months after the onset of symptoms — a choice that has no scientific basis.
Misinterpreted data
The article used public data on cases of severe acute respiratory syndrome (SARS) in Brazil from 2020 to 2023. The problem is that the system used to record this data was designed to monitor severe respiratory illnesses at the onset of the disease, meaning it is not the best for this type of research.
The system records dates such as symptom onset, hospitalization, discharge or death, and vaccination. Most of this information (except vaccination) is entered manually, which increases the risk of errors and requires adjustments to ensure accuracy.
Furthermore, the system only records deaths that occur during hospitalization for SARS or SARS-related deaths that occur outside the hospital. Because the system focuses on SARS deaths, the definition of “non-COVID-related” deaths is inherently flawed. The most appropriate database for this analysis would be the Mortality Information System (SIM), which records all deaths regardless of cause.
Figure 1: Evidence of misinterpreted data and recording errors
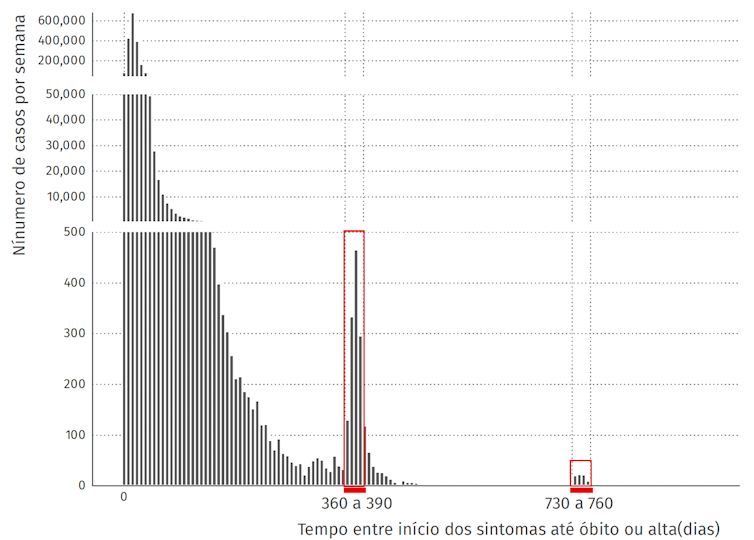
Figure 1: Number of cases in the database according to the time between onset of symptoms and death or discharge, in days. Each bar represents a 7-day interval. The red rectangles represent 360 to 390 days and 730 to 760 days.
Because the system was designed for acute cases, records with intervals over 3 months likely reflect data entry errors. Using the same database as the paper, we found that most cases had an interval of up to 14 days between symptoms and discharge or death, as shown in Figure 1.
Notably, about 99 percent of cases are between 0 and 84 days old. The number of cases with longer time intervals gradually decreases, until they reach intervals closer to one or two years. These spikes suggest errors in data entry, specifically in the year. A database of over two million cases, in which only 0.07 percent of cases have this long time interval, reinforces the idea that these records are errors.
Analysis errors and their impact on results
Despite these errors in the records, they are not expected to be concentrated in specific groups, such as vaccinated or unvaccinated. Thus, the results obtained from the analysis of these data should reflect general patterns in the sample. However, the study used an inadequate analysis.
The main problem is related to the timing of the start of vaccination in Brazil, which began in 2021. Therefore, cases from 2020 cannot be compared with vaccination, since there were no vaccinated people at that time.
The correct comparison would be between people with onset of symptoms in the same period of the year (calendar time). In this case, vaccinated and unvaccinated people will be compared under similar conditions of health system capacity and pandemic control measures. However, the authors compared these groups based on the interval between symptoms and death or discharge.
So, people infected in 2020 were compared, for example, to people infected in 2022, ignoring important differences. In 2020, the health system was unprepared for the pandemic and there was no vaccine available. In contrast, in 2022, vaccines were widely distributed and the number of new hospitalizations due to COVID-19 was significantly lower.
Another significant problem in the study is the way vaccination status is categorized. The SARS system links vaccination dates, but these can be recorded after hospital discharge, including doses administered after the onset of symptoms. This distorts the analysis, as these doses do not represent vaccination status at the time of infection.
The correct classification should only consider doses received before the onset of symptoms because doses received after the onset of symptoms refer to individuals who were not vaccinated at the time of infection.
Because the paper does not specify how vaccine doses were counted, we considered vaccination before or after symptom onset to be equal to try to match the 1/2/3 dose classification found in the paper. In our analysis, the numbers for the wrong approach are similar, but not the same, as those in the paper. All data and codes used in our analysis can be found here.
Figure 2: Impact of misanalysis
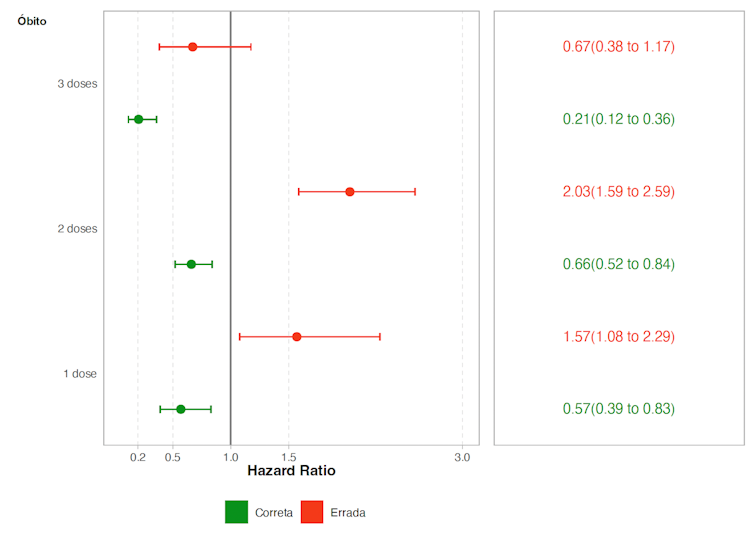
Figure 2: Hazard Ratio estimates (a measure that reflects the risk of death comparing groups with 1/2/3 doses versus unvaccinated individuals). Estimates were obtained using the correct model (calendar time) and the incorrect model (time since onset of symptoms). Values less than 1 indicate protection of that group in relation to unvaccinated individuals and values greater than 1 indicate increased risk of that group in relation to unvaccinated individuals.
The results using the correct and incorrect approaches are quite different. As shown in Figure 2, using the incorrect method generated a false association between vaccination and increased risk of death. In contrast, the correct analysis revealed vaccination as a protective factor.
The role of bad science in spreading misinformation
It is important to note that even using the correct approach (using calendar time), the type of analysis presented in the article is not ideal for answering the research question. The authors limited the sample to people who survived more than a year (or 3 months) after symptoms, ignoring deaths in the early period. However, it is necessary to assess the risk of death from the early period.
An illustrative example of this problem can be found in World War II when reinforcements for damaged aircraft were initially prioritized in areas with the most bullet holes. However, it was concluded that the opposite was true: if the aircraft managed to return, it was because these affected areas were less critical, and it was then decided to reinforce the areas with no apparent damage. Similarly, restricting the analysis of the article to survivors after one year distorts the results because it excludes cases that did not survive and that would be crucial for a complete analysis.
The article discussed here is being used as evidence to fuel anti-vaccine movements on social media. This backlash directly reflects the responsibility of the authors, reviewers, and editors. By failing to ensure rigorous analysis and accurate interpretations, they end up contributing to the spread of misinformation.
Serious errors such as those presented make any reliability of that study impossible. However, when it is accepted and publicized as valid, it ends up creating a false sense of uncertainty about the safety of vaccines and weakens public health efforts. When bad science spreads, it fuels pseudoscience and misinformation, becoming a real danger to public health. _______________________________________________________________________________________________
is a Research Fellow at the Department of Medical Statistics at the London School of Hygiene & Tropical Medicine; is President of ACB, Academy of Sciences of Bahia (ACB), and is a Researcher at Fiocruz and Professor at the Faculty of Medicine of Bahia, Federal University of Bahia (UFBA).
This article was originally in Portuguese and is republished from The Conversation.